Content
AI: On the advance worldwide, but not entirely transparent
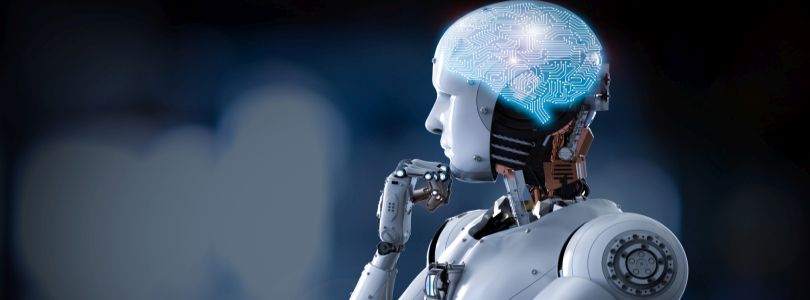
Interview on artificial intelligence
Dr. Josef Fleischmann, group leader and patent examiner at the DPMA in the field of data processing and information technology, on opportunities and risks in the development and use of artificial intelligence.
Why is everybody talking about AI these days?
Why is everybody talking about AI these days?The fact that artificial intelligence now even plays a role in "Tatort" films shows that the topic has arrived in the middle of society. The first research results in this field were already published in the 1950s. But it was only with the broad availability of powerful computers that could carry out computationally complex learning and training procedures that the AI received a new boost. At the same time, the amount of data collected in all areas of technology and society has increased enormously in recent years. As a result, the need for automated methods to evaluate this data has increased significantly.
What is so special about AI?
The fascinating thing is that this technology tries to imitate the functioning of the brain of a living being: Developers create models of neural networks and "train" them with data, for example to recognize objects in images or to automatically capture handwritten texts. In this way, computers can solve certain cognitive tasks that previously could only be solved by humans. With the decisive advantage that with appropriate scaling of computing power or the use of special hardware, much larger amounts of data can be evaluated than would be possible for humans. In addition, the analysis of a situation and the corresponding reaction to it take much less time.
How does this affect everyday life?
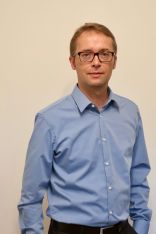
Dr. Josef Fleischmann
Take your smartphone: AI methods have become part of everyday life due to the spread of increasingly powerful devices. For example in the form of speech assistance systems or in the possibility of having faces of people identified and assigned in one's own digital images. "Smart" assistants in mobile phones try to provide their users with information that is appropriate to the current situation by making suggestions or offering help on the basis of recognized regularities.
What other application areas are there?
One of the important capabilities of AI systems is the recognition and classification of patterns and rules in data sets. This has resulted in popular applications such as the recognition of handwriting or the learning of games such as chess or Go. In recent years, AI methods have increasingly been used in image processing to recognize objects, for example in robotics, in autonomous vehicles or in medical diagnostics.
What further developments are to be expected in the near future?
The progress made in medical imaging makes it easier to understand the processes that take place in the human brain during certain mental processes, such as the timing of signals in communication between brain cells. These findings will then make it possible to model artificial neural networks more precisely. And these networks will probably be much more efficient than the current approaches.
What are the disadvantages or risks?

In my understanding, one disadvantage is that methods such as deep learning with neural networks are currently very widespread and successfully used, but the theory behind them is not yet fully understood.
An example: An AI indicates that an animal image is 90% likely to be a cat. However, we do not know what criteria the AI uses to come to this conclusion; we do not know what it believes to see. This question is therefore a subject of research.
So in a way, AI is a kind of "black box"?
To a certain extent, yes. In this context, the phenomenon of so-called adversarial examples, which is fascinating in a certain way, is often discussed. In such a case, for example, an image to be judged is slightly modified in "bad" intent. This leads to the fact that the AI recognizes a completely wrong object on it, while a person looking at the changed picture usually cannot make out any difference at all to the original.
So there is obviously still a lot of research to be done here.
Yes, science wants to make the decision-making process in neural networks more transparent. One wants to go from a "black box" system to a "gray box" system, in which one can at least partially make transparent why an AI system makes one decision or the other.
Do you see any other risks?
Another disadvantage could be seen in the fact that the current methods of training increasingly complex neural networks with large amounts of data require enormous computing effort. There are therefore concerns that only the really large IT companies, which have enormous computing and storage capacities and financial resources at their disposal, will be in a position to offer high-performance AI solutions in the future.
Further articles in this dossier:
Pictures: iStock.com/phonlamaiphoto, J. Fleischmann, iStock.com/wigglestick
Last updated: 9 December 2024
Social Media